Brayden Binder ’22: Obstacles
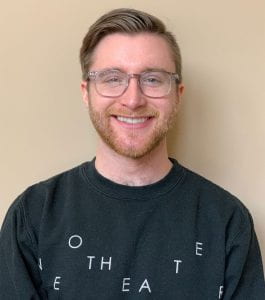
Hello again everyone! If you haven’t read the first blog post, you should go and read that because this one will talk specifically about my project with all the obstacles I ran into and my plan moving forward. Just a quick refresher though, I am Brayden Binder, a rising-senior Business Economics major and Finance minor who performed growth theory Economic research for Providence College over this summer.
I relied heavily on the expertise of my mentor, Dr. Fang Dong, throughout the entire research process. A big change from the Economic Capstone class research was that the writing and editing processes with my summer research were not as linear. When I would read a new academic Economic journal article, I would see if I could fit it in somewhere in one of my paper’s sections or hold it for later. Thus, I restructured my academic literature review section quite a few times, just to make sure it had a nice flow to it and incorporated what I wanted to say. My interpretation or analysis of many studies however, was lacking when the study used high-level models or estimation methods. It happened several times that I would write down a finding from a paper, check it with Dr. Dong, and my interpretation would be somewhat off.
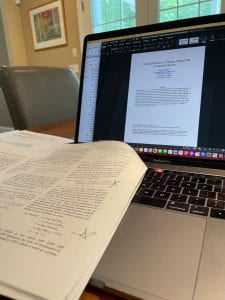
Thus, although my Capstone class and Intro to Econometrics classes prepared me well to be able to read the Economic works, some studies were much higher level than I was used to. Of course, this choice of reading higher-level papers was done on purpose, as I knew that I wanted to contribute something to the growth theory field. To make it so I contributed something, I needed to establish my findings within the framework of past studies and state where my work fits in. Due to this, I had to ask for help from Dr. Dong a lot while reading many papers to check that I was not misconstruing big ideas or even small details that I included in my work. This was the first big hurdle for my paper: learning how to interpret the higher-level economic jargon that was sometimes used.
After I was able to better understand some of the language higher-level Economic researchers sometimes use, my literature review section improved greatly, and I was able to move on quickly to data collection. Thankfully, the article from which I based my model off had listed their data sources, so I had a good idea where to look. Oddly enough, even though I had a blueprint for where to find the data for my variables and the methods used in the creation of them, this stage took the longest and was by far the hardest. Instead of using the main data source from that paper, I used a more Economic oriented source, the Penn World Table.
This problem I encountered may be the hardest to explain, as people without Stata experience may not understand what the issue really is. Fundamentally, to make the model you are using work and be accurate, you need to have your data line up in terms of datapoints per year and the countries being looked at. For instance, if your data looks at 50 countries, but one variable is measured 4 times a year, and another once a year, your model will suffer and not be accurate. You can have holes in your data, but to make your model the best it can be and to have the most accurate results, you need to make sure your raw data is in order.
The issue I ran into was massive and threatened the possibility of publication in the future. There were so many times where my data did not line up, and sometimes I could not do anything about it. If you remember the definition of human capital being “the relative skills and knowledge of a population”, you can see the vagueness of how this can be measured. There are so many ways the human capital stock of a population can be measured, and these different human capital metrics can be collected quarterly, yearly, in 5-year intervals, and more.
If you think about the data collection experience like building a pyramid, you need to first establish the years and countries you have data for and work up from there. This foundation of the years aligning needs to be set before any work is done, and usually when collecting Economic data, the default number of times this data is collected is once per year. As said before, this is not the case for human capital metric data. Thus, I had to ask for so much help from Dr. Dong, with so many long Zoom calls, as there was no way I could do this work on my own. She was instrumental in figuring out ways to combine data that was sometimes collected yearly, in 3-year intervals, and more down into a single dataset. Sometimes, it was impossible for the years to match up, and I had to throw out a specific metric completely. This was a huge bummer, as I had a set number of metrics I wanted to use with specific sources.
Thus, it took a while to even have the foundation of my metaphorical pyramid created before I could begin doing any work with the models. In short, I spent a very long time searching for data that was reputable and could fit in my work. This forced me to edit what I said the paper was examining so many times, it also caused me to question the main ideas and purposes of the paper. I am so thankful for Dr. Dong, as the work she did would have taken me most of the 10 weeks to finish. This second main issue was much bigger than the first, as there is no way I am writing a paper without any usable data.
I encountered one final large obstacle regarding the actual results of my model, which I will discuss in my third blog post alongside the future of the paper for publication.